CAIML Seminar: “Evolutionary Implications of Multi-Scale Intelligence”
Seminar talk by Benedikt Hartl on November 27, 2024.
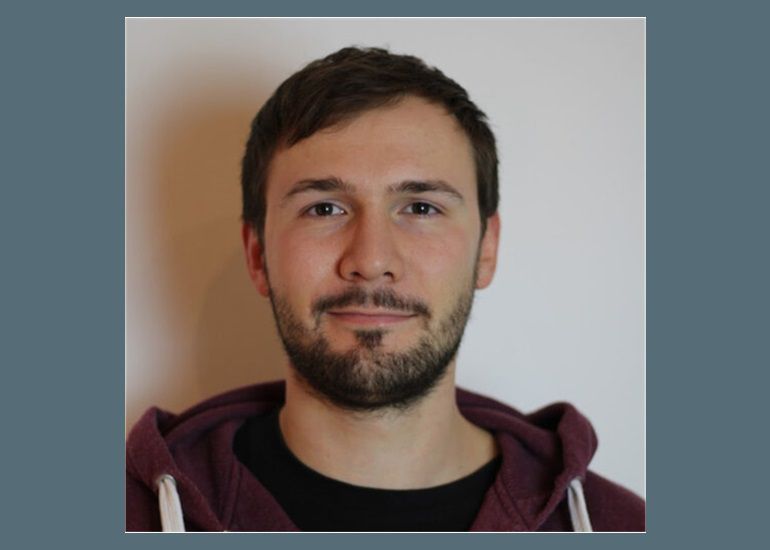
November 27th 2024
- 11:00 – 12:00 CET
- TU Wien, Campus Gußhausstraße, EI8 Pötzl Hörsaal
-
1040 Vienna, Gußhausstraße 27-29
Block CD, Ground Floor, Room CDEG08
CAIML Seminar with Benedikt Hartl will take place on November 27, 2024 in EI8 Pötzl Hörsaal. The seminar talk will be based on this paper.
Abstract
The scientific community increasingly acknowledges the complex Multi-scale Competency Architecture (MCA) of biology [1]: This architecture consists of nested layers composed of competent agents that form a self-organizing substrate for the layers above while relying on the adaptability of the layers below. Understanding how natural selection gives rise to such architectures remains a fundamental question in evolutionary biology.
In this talk, we present insights from in silico morphogenesis experiments, exploring how the collective decision-making competencies of MCA components influence evolutionary dynamics. To this end, we model morphogenesis - self-organized pattern formation - using Neural Cellular Automata (NCAs) [3]. NCAs simulate unicellular agents that perceive their local environments on a two-dimensional grid and update their states based on decisions made by internal, trainable neural networks. These networks act as proxies for biological intracellular regulatory pathways. We aim to train these agents to collectively self-assemble a two-dimensional target pattern by regulating their cell states on the NCA grid. We achieve this by employing evolutionary algorithms to optimize the neural network parameters of the unicellular agents.
To investigate the impact of different levels of component competencies on evolutionary dynamics, we systematically vary the reliability of the agents to regulate their states during development. This variation allows us to continuously scale the unicellular competency levels from an MCA to a direct encoding scheme that lacks intrinsic problem-solving abilities. Our results demonstrate that an MCA-based evolutionary process operates significantly more efficiently (on average) on noisy self-orchestrated pattern formation tasks compared to direct encoding schemes. Furthermore, evolved NCAs demonstrate strong generalizability to changes in system parameters and adaptability to modified evolutionary objectives.
These findings suggest that the cellular problem-solving competencies inherent to MCA systems profoundly influence evolutionary outcomes. They highlight the evolutionary advantages of multi-scale collective intelligence, suggest broad implications for understanding the emergence and robustness of biological complexity, and offer new insights for designing adaptive artificial systems.
[1] M. Levin, “Darwin’s agential materials: evolutionary implications of multiscale competency in developmental biology”, Cell. Mol. Life Sci., 80, 142 (2023), https://doi.org/10.1007/s00018-023-04790-z
[2] B. Hartl, S. Risi, and M. Levin, “Evolutionary Implications of Self-Assembling Cybernetic Materials with Collective Problem-Solving Intelligence at Multiple Scales”, Entropy, 26, 532 (2024), https://doi.org/10.3390/e26070532
[3] A. Mordvintsev, E. Randazzo, E. Niklasson, and M. Levin, “Growing Neural Cellular Automata”, Distill (2020), https://doi.org/10.23915/distill.00023