Univ.-Prof. Dr. Stefan Szeider
Algorithms and Complexity Group (192-01), Institute of Logic and Computation, Faculty of Informatics,TU Wien
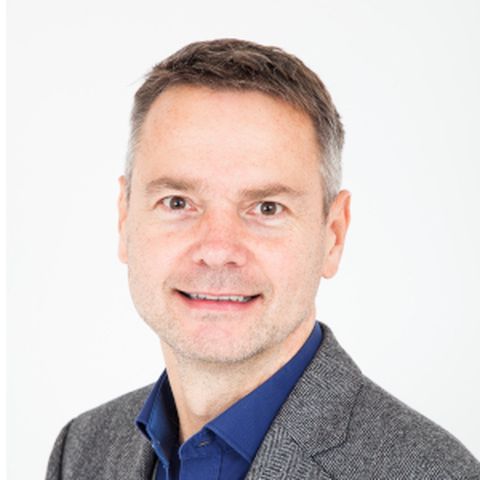
On This Page
Short Bio
Stefan Szeider is a full professor at the Faculty of Informatics at the Vienna University of Technology (TU Wien), Austria. He chairs the Algorithms and Complexity Group and the Vienna Center for Logic and Algorithms. Before that, he worked for several years at universities in Canada and the UK. He is the first Austrian Computer Scientist who received a grant from the European Research Council (ERC Starting Grant 2009). Szeider’s research focuses on developing and analyzing efficient algorithms for problems that arise in Artificial Intelligence and Automated Reasoning.
Prizes, Awards, Memberships
- Invited long-term visitor at the Simons Institute for the Theory of Computing, Berkeley, programs “Satisfiability: Theory, Practice, and Beyond” and “Theoretical Foundations of Computer Science”, Spring term 2021
- Best Paper Award at CP 2020 (with Tomas Peitl), “Finding the Hardest Formulas for Resolution”, the 26th International Conference on Principles and Practice of Constraint Programming
- First prize in the PACE 2019 competition (with André Schidler), the 4th Parameterized Algorithms and Computational Experiments Challenge
- Chair of the MSCA doctoral program “Logics for Computer Science”, (LogiCS@TUWien)
- Founding Co-chair of the Vienna Center for Logic and Algorithms (VCLA)
- European Research Council (ERC) Start Grant “The parameterized Complexity of Reasoning Problems”, 2009
- Chair of the Jury for the Heinz Zemanek Dissertation Price of the Austrian Computer Society
- Journal of Computer and System Sciences, Associate Editor
- Journal of Artificial Intelligence Research, Editorial Board Member
Research Interests
Szeider combines algorithmic and logic-based methods to solve hard computational problems in Artificial Intelligence, Automated Reasoning, and Combinatorial Optimiziation. Recently he proposed logic-based methods for learning fast-inference Bayesian networks and succinct decision trees from data.